Media Query Source: Part 46 - CIO (US digital magazine); 10 key roles for AI success (2024 update)
- CIO (US digital magazine)
- 10 key roles every AI team needs
- Increased criticality of data engineer role
- Data modeler role was missed in first piece
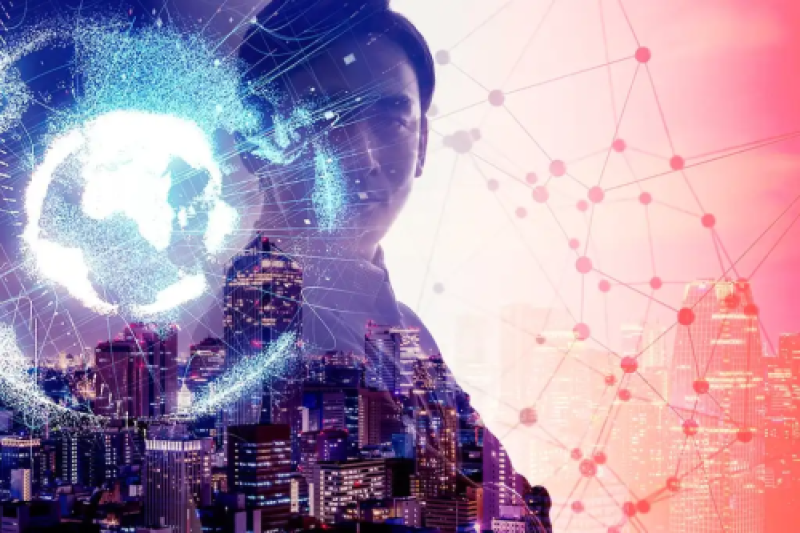
My responses ended up being included in an article at CIO (October 17, 2024). Note that CIO overwrote the original June 7, 2022 article with this updated content. Above image from cited article.
The query responses I provided to a media outlet on September 23, 2024:
Media: Erik – About a year ago, [we] spoke to you for a story about the 10 key roles for AI success:
https://www.cio.com/article/400380/10-key-roles-for-ai-success.html
I've been asked to update this story – and the world has shifted quite a bit since then! Could you please review these quotes and let me know if anything has changed. Does this role still matter? Are there other roles that should be added to the list?
Media: a quick correction – the story was from two years ago, not one! LOL That's a HUGE difference given what happened at the end of 2022!
Previous quotes:
Data engineers build and maintain the systems that make up an organization’s data infrastructure. They are crucial to AI initiatives because data needs to be both collected and made suitable for consumption before anything trustworthy can be done with it, says Erik Gfesser, director and chief architect at Deloitte.
Gfesser: My statements here are still viable. With respect to AI success, the role of data engineer largely hasn't changed over the past two years, and the criticality of this role continues to increase: without data engineers, AI initiatives will simply grind to a halt.
However, I've greatly simplified my definition of "data engineering" over the past two years by describing it as software engineering that focuses on data as the product.
Why have I done this? Because the core goal to provide data to downstream consumers such as AI remains the same, regardless of whether all data engineering tasks apply to all downstream consumption use cases.
Sometimes it seems that data engineers aren't needed because of the mode of this downstream consumption.
For example, when an LLM prompt is issued it seems as though queries are directly hitting raw data, but queries are really hitting a model already trained on vast amounts of raw data, with results based on statistical relationships between words and phrases.
“Data engineers build data pipelines to collect and assemble data for downstream usage, and in a DevOps setting, they build pipelines to implement the infrastructure on which these data pipelines run,” he says.
The data engineer is foundational for both ML and non-ML initiatives, he says. “For example, when implementing data pipelines in one of the public clouds, a data engineer needs first to write the scripts to spin up the necessary cloud services which provide the compute necessary to process ingested data.”
Gfesser: My statements here are still viable, as well. However, the tooling used by data engineers continues to mature, and the community of data engineers continues to expand, making common data pipeline patterns more widely known.
Programming assistants have become prevalent, but these still need to be used with caution because the models behind these assistants are still based on statistical relationships that don't understand the code they generate.
And because these models don't understand their output, the bearing continues to be on data engineers to ensure that what is being generated is appropriate to be used within a given code base.
From my experience, the one area of data engineering that continues to require the greatest human touch is data modeling. In other words, the structuring of stored data to cater to the needs of downstream use cases, especially those dependent on exact results.
Media: Are there other roles that should be added to the list?
- Data scientist
- ML engineer
- Data engineer
- Data steward
- Domain expert
- AI designer
- Product manager
- AI strategist
- Chief AI Officer
- Executive sponsor
Gfesser: Adding roles can be tricky, because roles aren't standardized in industry, and some roles cover a wide variety of tasks. The original article, for example, mentioned that the data scientist role is really a mix of roles.
If I were to call out an additionally needed role missing from the original list, it would be the data modeler role. While I've often seen data engineers serve in this capacity, it's important to recognize the fact that data modeling is a task carried out in collaboration with some of the other roles, especially the domain expert and the first three roles listed here. While the data engineer focuses on data as the product, data modeling is a specialized skill that I've often seen data engineers (as well as the other aforementioned roles) lack, and as such I think it deserves to be called out as a distinct need.
Note to reader: the above quotes of me cited by CIO aren't direct quotes. My original query responses can be found at the following location:
https://erikgfesser.com/media-query-source-part-40-may-6-2022-ten-key-roles-every-ai-ml-machine-learning-team-needs-data-engineer/